Artificial intelligence as an anti-corruption tool
The term artificial intelligence (AI) covers technologies where machines mimic human intelligence to solve complex problems. On one side we find methods where an algorithm, a ‘recipe’ on how to handle a specific set of inputs, drives the computing process that determines or suggests an output. Machine learning (ML) resides in this domain, where multiple methods of various levels of complexity are applied to solve different kinds of problems. Some of these techniques need a dataset to ‘train’ the algorithm on how to handle the information. Algorithmic bias is often inherited from the datasets used to train the algorithm. Some systems ‘learn’ how to achieve the optimal result with no supervision. Artificial neural networks mimic the way our brain is constructed. Millions of calculations are performed and sent between the nodes of the network, generating complexity that can become impossible to explain. The ‘black box problem’ refers to opaque calculations in complex algorithms. More advanced imitations of human intelligence, artificial general intelligence (AGI) or superintelligence, still belong in the future, and is not a focus of this document. Neither shall we address robotics.
In our highly digitised societies, artificial intelligence is embedded in gadgets, cars, and consumer goods. Algorithms calculate what music you should listen to based on your previous choices. Machines suggest which book to buy or who to invite for a date. Translation tools are rapidly improving. Algorithm driven chatbots reply to our questions in text or spoken language.
The technology is affecting more and more parts of life in sufficiently digitised economies with a certain level of e-government, where transactions or interactions with authorities to a large extent are digital. Automated decision systems are controversial, but still deployed in social security programmes, within the legal sector, in policing, insurance and security.
AI and machine learning are also applied to uncover or detect money laundering. Tax authorities use AI to predict risk for tax evasion, or to monitor and identify suspicious tenders or bids in public procurement. Transparency International UK is adopting AI-solutions to automate searches in public records and strengthen its analytical capacity.
However, some applications of AI and automated decision systems in society remain controversial. Questions persist on how to handle biased algorithms, our ability to contest automated decisions, and accountability when machines make the decisions. How such systems relate to the right to privacy, the right to explanation, and the ‘right to be forgotten‘ also remain topics of debate. Nevertheless, due to the efficiency, apparent neutrality, stable performance, and cost savings associated with AI based processes, such tools are likely to be applied in more and more areas in the future.
High hopes in development
Development agencies express hopes to the promises, and sometimes deep concern over the pitfalls, embedded in these technologies. Projects in which AI plays a key role in anti-corruption, or where digital processes or mobile technologies are drivers for new ways to do business, are discussed below. Some designs are introducing novel, digitised procedures omitting previous corruption-prone tasks. Other projects have a ‘direct’ approach to reveal previously hidden transactions or actors in fraudulent behaviours.
Digitised interactions between society and its citizens are in many cases the foundation on which to build AI applications. Redesigning the ways of doing business or governance to enable automation and the assistance of AI may in some cases also reduce the risk for fraud or corruption.
Some fear that AI and automation of processes will lead to the loss of valuable jobs, in particular in developing countries. Jobs lost to automation are in digitised societies replaced by jobs demanding higher or different skillsets. One challenge is therefore to strengthen the education system. Another challenge in developing countries is the lack of consistent, digital, reliable data.
Reforms are needed in education, governance, and the legal sector to take advantage of the fourth industrial revolution. Some researchers have argued that only a handful of countries in Africa are able to exploit AI to advance sustainable development. Technology-driven businesses such as Uber, where AI is at the core of its operations, are present in several African countries. The term ‘uberisation’ is derived from the name of the company and characterises peer-to-peer transactions with the aid of a digital platform. Such transactions bypass corporations as the organising body of the workforce. Becoming self-employed contractors is the new norm. Affordability and the spread of internet and mobile connection will be an important driver for future AI development and digital governance. In May 2019, Uber drivers in several countries went on strike due to decreasing average wages and the company charging them increased commissions.
AI applications have been pilot-tested to identify risk of corruption or fraud in public procurement in Mexico and Ukraine. South African tax authorities are looking into possibilities of utilising AI, and India is discussing options to monitor social media to spot possible tax evasions. However, some of the solutions are questionable from an ethical perspective.
How and by whom the technology is introduced in developing countries should gain attention and possibly trigger debate. Enterprises such as DeepMind, Google, Facebook, and IBM are establishing AI-driven projects and research institutions in the Global South. This might lead to increased interest in investment and applications of AI within sectors such as health, agriculture, education, and production. Several agents emphasise the importance of research happening on site, where the technology is to be applied. The hope is that local ownership of the knowledge and tools will reduce the effect of a new, digital colonialism where multinational corporations own the data and therefore can profit from its exploitation.
Some of the projects, institutions, and reference documents identified through this study are collected in an online knowledge base. The dataset contains links to projects, companies, and institutions in the field of AI related technologies such as blockchain or big data. The entries are not restricted to applications within the fields of anti-corruption, since technology applications used in other areas in society might have solutions suitable as models for possible anti-corruption projects.
In a roundtable session during the 2019 OECD Global Anti-Corruption & Integrity Forum, we briefly presented our preliminary findings. More important, however, was the opportunity to receive feedback from a wide array of stakeholders during the forum. Feedback from the sessions and individual meetings are to a certain extent included in the following sections.
Uncovering corruption and fraud with artificial intelligence
Oxford Insights lists Artificial intelligence as ‘the next frontier in anti-corruption,‘ partly due to its ability to reveal patterns in datasets too large for humans to manage. Applying AI to detect elements of interest, humans can focus on details and follow up on suspected misuse, fraud, or corruption. Mexico is an example of an economy where AI tools alone might not be sufficient to win the battle.
During the last decade several reforms in Mexico have been carried out to stimulate economic growth and address high levels of corruption. The Tax Administration Services of Mexico piloted a project to detect fraudulent operations among taxpaying companies using AI algorithms and analysis tools. ‘Within three months of a six months pilot scheme, 1200 fraudulent companies were detected, and 3500 fraudulent transactions identified. The identification and analysis of these irregular activities would have taken an estimated 18 months of work without the use of AI,’ says the report Towards and AI Strategy in Mexico, authored by Oxford Insights and C-Minds and initiated by the British Embassy.
Many areas of the Mexican economy have seen progress, including the telecoms sector. Telecom was previously dominated by one player and is now open for competition. This has led to a significant reduction of connectivity cost, and the country is now preparing for its highest investment ever. The goal there is to deliver 4G mobile connectivity to more than 90% of the population within 2024. Affordable connectivity is key in a society working towards digital government services.
The next step is to develop an AI strategy for the country. Suggestions such as working towards AI-based solutions to deliver government services for less or to introduce AI driven smart procurement will be included in the forthcoming national AI strategy. In short, Mexico has ambitions to be among the first ten countries in the world to commit to a national strategy for AI.
Digital tools and artificial intelligence have also been applied in pilot projects to uncover fraud and tax evasion in public procurement. The Mexican Institute for Competitiveness (IMCO), together with Participatory intelligence (OPI) used automated queries (AI) on millions of data records to analyse the government’s contracting procedures to identify corruption risks. The dataset covered public procurement from 2012 to 2017 and contained 6 million data lines, with 230 million cells from 9 different sources. Using the evidence from that project, they built a Corruption Risk Index, identifying risk in the more than 1500 buying units studied.
Investigator Fernando Alcázar concludes, ‘One of the most important challenges in the fight against corruption begins with the readjustment of the public procurement policy of the State. If we really intend to use public spending as an enhancer of our development, the task starts here.’ Mexico ranks high among OECD countries to implement open government data. The dataset used to identify corruption risk is also shared in public. And the country is the first to embed the project ‘Open Up Guides,‘ introduced in 2015 byTransparencia Mexicana as an official standard.
Anti-corruption laws, regulations, and anti-corruption institutions are in place in Mexico. However, the enforcement of laws is not, according to recent U4 research. A key problem is the lack of will or ability for law enforcement to react to the corruption once it is uncovered. During the last five years, the country has seen its ranking on the Corruption Perception Index (PCI) drop (lower scores reflect higher levels of corruption).
South Africa evaluating AI as a tool to monitor tax compliance
In its 2018/19 Annual Performance Plan, the South African Revenue Service (SARS) states that the service will investigate the use of AI and the latest analytics tools to gain a ‘better understanding of taxpayer compliance and behaviours.’ With enhanced data analytics capacity, the service hopes for better decision-making without releasing details on specific aims at this point. A collaboration between several financial and government institutions in SA is also drafting a policy proposal evaluating the regulation of crypto assets and entities providing the digital currency. At this stage the aim is to register the actors and to gain insights into this market. At a later stage the authorities will evaluate if ‘crypto asset activities could fit into existing regulatory frameworks.’
India planning to use AI to compare social media ‘flashing’ and tax records
In 2017, the Union Finance Ministry, India launched ‘Project Insight‘ to monitor high value transactions, including monitoring social media accounts, to detect spending patterns and to compare these with tax records. Project Insight is utilising AI technologies for monitoring transactions and possibly scanning social media accounts. The Union Finance Ministry entered into a contract worth US$100 million with L&T Infotech (Larsen & Toubro) to help with Project Insight. Critics accuse the project of being both costly and violative of individuals’ privacy.
In 2016, the government undertook related efforts, including ‘Operation Clean Money‘, which removed small notes from the Indian currency, as well as updating the ‘Benami Transaction‘ acts. The government set out to reduce tax evasion by introducing new technologies, but also by taking out outdated elements. Removing the small banknotes was aimed at reducing the black economy.
Revealing fraud in public procurement
Several initiatives have been launched in different countries to combat corruption and fraud in public procurement. Open contracting and transparent procurement processes are seen as important steps towards a solution. High hopes were connected to Prozorro, the Ukraine open source e-procurement system, launched in 2016.
The State Audit Service developed 35 risk indicators to help evaluate which tenders were in need of closer inspection. But it turned out that as soon as these indicators were known, fraudulent bidders adapted to that fact and then took action to avoid audits. Transparency International Ukraine therefore launched their own software, ‘Dozorro,’ based on machine learning and trained to identify tenders with a high risk of corruption. The new tool is not bound by pre-defined indicators or formulas. ‘As soon as experts and activists see that new “trickery algorithms” have emerged, the system will automatically adjust,’ the developer Volodymyr Flionts explains. The system will dramatically increase the efficiency of expert analysis of tenders due to its ability to scan large amounts of data in a short period of time. When illegal or suspect tenders or purchases are discovered, they are reported to the authorities to be investigated. Without the capacity of AI tools, a continuous monitoring of the bids and tenders would have been impossible. As a result, Ukraine has improved its position on the PCI index during the last three years.
Machine learning tool indicating probability of corrupt civil servants
The Brazilian Office of the Comptroller General has developed a machine learning application to estimate risk of corrupt behaviour among its civil servants. By entering the social security number of a civil servant into the dashboard, the app returns the probability of the person being corrupt, displayed on a simple gauge. Hundreds of variables are included in the analysis performed by the tool, such as how the person was employed – by political affiliation or education. Possible criminal records, business and shareholder relations, political affiliations, and level of position are all included in the calculation. A large dataset on convictions of civil servants was used to train the algorithm.
A similar system is now developed to identify the risk of a company being corrupt. The main challenge now, according to Thiago who is leading the group, is that the Brazilian law does not permit any sanctions either against individuals or businesses. No investigation can be started on the basis of this tool, and no bids from one of the high-risk companies can be refused.
Paperless governments and public declarations of interests
Argentina declared themselves a paperless government on January 1, 2019. The ambition is to reduce bureaucracy, speed up government service, reduce costs and fight corruption. ‘Technology is becoming a key ally in the fight against corruption,’ writes the Director for Digital Innovation in Government, Carlos Santiso, in a WEF article. Digital processes are fundamental to the application of AI-enabled tools to reveal or predict corruption. The lack of law enforcement to handle the uncovered crimes is the next obstacle to overcome.
Similarly, from an easily navigated dashboard called InfoProbidad, citizens in Chile can monitor the declarations of interests and business assets of those in high positions in public service. The mandatory registration was established by law in 2016 and contains to date nearly 40,000 statements. In addition, the register contains information on personal property, real estate, and family relations. It further contains building permits and public procurement contracts. The register is managed by the Chilean Transparency Council and is meant to serve the media, non-profit organisations, and the general public. As shown below, Brazil has taken such registers a step further.
Early warning on future corruption in Spanish provinces
A Spanish research project has shown how AI and neural network analysis of historical data on corruption can be applied to uncover and even predict future risks of corruption. Analysis of several datasets and the relation between them has led to new understanding of the risks for corruption that were nearly impossible to uncover by manual processes. The researchers found that a combination of factors such as real estate taxation and increase of prices, economic growth, growing numbers of deposit institutions, and the same political party remaining in power for a long period of time seemed ‘to induce public corruption.’ The researchers claim it is possible, with the help of AI, to predict the risk of corruption in public procurement, years before it happens.
Digital reports on development aid
Corruption and fraud in donor portfolios represent one challenge where new technologies may speed up investigation or ease the detection of suspicious incidents. The OpenAid concept by International Aid Transparency Initiative (IATI) has been around for a while, and has been adopted by several countries. For AI tools to be efficient, transactions and reporting need to be harmonised. Development projects across several countries, involving multiple languages, currencies or reporting procedures may need ‘cleaning’ before an AI application is able to monitor efficiently enough to detect possible irregularities with a satisfying level of precision. The following example stems from a highly digitised donor organisation, where the structure is already in place. Still, the reports need a human review before they are shared. To do this, a machine learning application is created to assist.
AI assisted review of reports to donors
Thousands of small and larger projects deliver quarterly reports on the progress of their work to ensure their credibility towards the donors linked up with GlobalGiving. An in-house team manually reviews all reports before they shared with others. GlobalGiving is among the largest crowdfunding communities for global non-profits. With more than 22,000 projects in 170 countries since the start, the review is a major effort. The organisation applies supervised Machine Learning and Natural Language Processing (NLP) to assist the review of thousands of reports from beneficiary organisations. The quarterly reports are reviewed to ensure a satisfying format before they are shared with subscribing donors for each project. The AI is applied to detect particular keywords and to clean the reports to remove ‘garbage.’ The AI-assisted review has more than halved the person-time needed to verify the quality of each report. GlobalGiving is a highly digitised organization and has raised close to US$400 million from nearly 1 million donors since the start in 2002. Using automation and AI to optimise and assist the review process has been important to save personnel time and administrative cost.
Mapping roads and monitoring road conditions – no maps no money
The World Bank collaborates with DevelopmentSeed in mapping roads using satellite imagery, mobile data and telemetry in Vietnam and the Philippines. Machine Learning is used to detect roads from satellite images and extract the information to maps and to a database driving an information dashboard. Countries around the world spend significant funds on roads without access to reliable roadmaps. The project aims at providing not only the maps, but also traffic data and information on the conditions of the roads. The system can be used to verify that roadbuilding and maintenance actually happens. Similar techniques are used for mapping high voltage lines where the World Bank wants to reward updates to the grid. Mapping the existing grid supports decision making and verification of the existence and whereabouts of the power lines.
Disputed partner for AI-driven monitoring of aid
The World Food Programme (WFP) announced early in 2019 a collaboration with the software company Palantir to streamline WFP’s information flows covering food- and cash-based aid. The collaboration will integrate data covering 92 million aid recipients in 30 different data systems. Palantir already has contracts with several US state agencies, including the FBI and immigration authorities.
Algorithms applied to track transactions and the location of recipients will flag unexpected behaviours, transactions or movements. By this the WFP can uncover attempts of fraud or misuse. Severe criticism has risen on how a company closely related US state security agencies are to develop data systems for an UN agency. WFP are handling very sensitive personal information about the most vulnerable communities. The fear is for possible inhouse leakage where sensitive information from this project will seep into other Palantir projects. WFP claims that no Personal Identifying Information (PII) will be handed over to the contractor.
Privacy International and ICRC has developed guidelines on the handling of sensitive data when using new technologies. The new tools contain great promises, but also huge risks for breaking the ‘do no harm’ principle.
‘The key to impact is being able to deploy analytics and technology in a business-specific way and to embed them organically into business processes, which in turn often have to be fundamentally reshaped to take advantage of new tools.’ (The new frontier in anti-money laundering).
Changing a faulty system using AI rather than chasing corruption
Rather than replacing bureaucrats with machines and keeping structures, there is an option to rebuild and simplify procedures in government services as digital solutions are introduced. Estonia is an example of a society gone digital in a short period of time. Some say that part of the reason for its achievements was the need to rebuild its institutions after they left the Soviet Union. Noteworthy is also the Estonian focus on its digital industry.
An example from Kenya shows a long-term project with the aim to stimulate foreign investments and local entrepreneurship by making it easier to establish a formal business.
IBM’s bid to bring Watson (the AI-computer that won Jeopardy) and cognitive computing resources to Africa was launched in 2014 in a plan to invest US$100 million over ten years. The cognitive technologies embedded in Watson are utilised to learn and discover insights from Big Data ‘and develop commercially viable solutions to Africa’s grand challenges,’ says the press release from the launch.
In 2013 Kenya was ranking as the 136th of 189 countries on the World Bank’s Ease of Doing Business Ranking. The score is calculated based on the country’s performance in areas such as procedures to start a business, interacting with authorities on construction permits, registering property, or paying taxes. One of the projects where IBM research became engaged was to simplify the process to establish a business in Kenya. ‘With our marching orders from the President himself, we embarked on the purest of the scientific method: collecting data – all the data we could get our hands on – making hypotheses, creating solutions, one after the other,’ says Charity Wayua in her TED presentation of the project.
The researchers and advisors started by identifying needed changes within the Kenyan legal system, their procedures, and regulations. The project simplified procedures and reduced interactions with the authorities from 11 steps previously required to start a business to just three. Not only has the revision made it easier to start a business, but the administrative cost of doing so has also been dramatically reduced. Each year since the project started, Kenya has improved its score. The goal to be among the top 50 countries in the world is within reach, as Kenya was listed at 61st in the 2019 ranking. The researchers are continuing to study how AI and blockchain technology may further ‘improve the efficiency and effectiveness of government service delivery.’ The efforts to climb up the Ease of doing Business ranking is not reflected in the corruption perception index. Kenya has climbed from a score of 25/100 in 2015 to 27/100 in 2018, where a higher score is an indicator of less corruption.
Hopefully, the consequences are reviewed on a broad scale as it develops. Other countries have experienced the pitfalls of allowing artificial intelligence systems take over governance without critical oversight. This is discussed in further detail below.
AI Strategies Heat Map
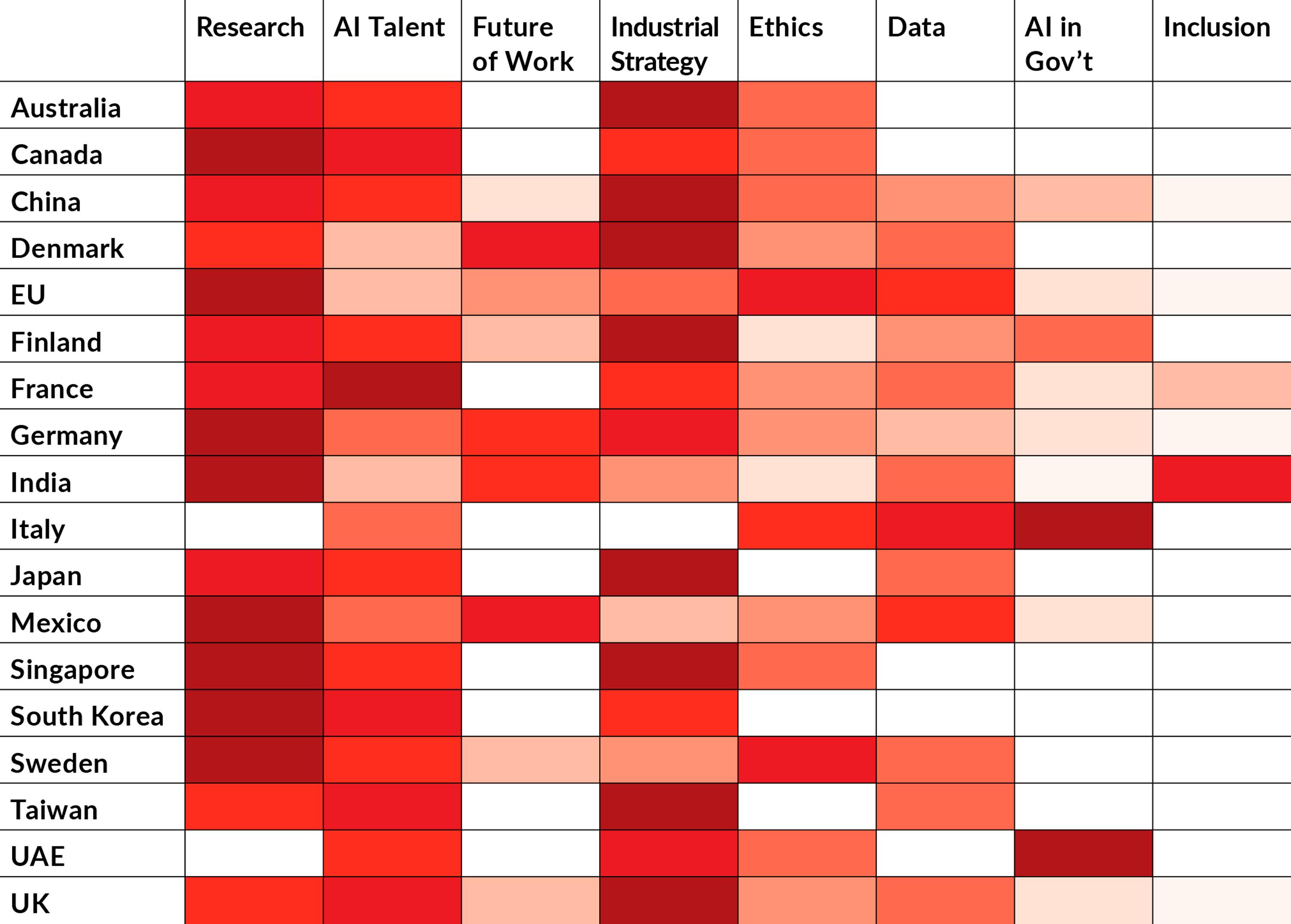
The CIFAR report shows the priorities of AI strategies in 18 states and regions that have implemented and dedicated funding to their implementation. The darker colors indicate which sector has gained most funding in each country and region.
Table from CIFAR 2018, Building an AI World: Report on National and Regional AI Strategies
The Canadian Institute for Advanced Research (CIFAR) released in late 2018 a report on national AI strategies developed around the world. Eighteen countries matched their criteria for having created ‘a set of coordinated government policies’ with a clear objective to exploit the benefits and minimise the cost of implementing AI in society. (The Future of Life Institute has published a similar list.) CIFAR analysed the main focus areas of the strategies and identified eight comparable themes. Even if they don’t share the same strategic priorities, industrialisation is the top priority among almost half the national strategies, closely followed by the development of research as a strategic goal. The assessment is based on the amount of funding directed to each area.
More data than ever – yet still a lack of data
Mobile technologies have been adapted with success in developing countries, given financial inclusion to millions, and given internet access and connectivity to many more. This has inspired a new wave of technology optimism for the introduction of artificial intelligence applications to solve severe challenges in developing countries. Expected to revolutionise health services, farming, industry, governance, and maybe even reduce corruption, some have high hopes and rarely emphasise possible threats.
The AI researcher Andrew Ng and former president of the World Bank,Jim Yong Kim, sat down for a Californian fireside chat in October 2018. They suggested that AI may become the ‘trampoline’ by which developing countries jump the development ladder rather than climb it. A prerequisite is that governments develop education systems, facilitate business opportunities, and strengthen governance to back up collaboration between these players in society. Andrew Ng promised to introduce his Stanford students to the most serious challenges of the world, to see what AI solutions they may come up with to solve them.
Others are less optimistic, claiming that the lack of rich and reliable data as well as challenges connected to infrastructure, education, and governance will prevent many developing countries from taking part in the fourth industrial revolution and therefore those countries will be left behind. Alexandra Gadzala from the Africa Center of the Atlantic Council claims that only Kenya, South Africa, Nigeria, Ghana, have the digital capacity needed to deploy AI applications on a broad scale. Other countries need reforms ‘in the areas of data collection and data privacy, infrastructure, education, and governance’ to benefit from the new tools.
Predictions described in the 2016 World Bank report on Digital Dividends estimate that as much as 60% of all jobs are ‘susceptible to automation in the developing world.’ The figures are even higher for some countries – frequently cited is the statement that 85% of jobs in Ethiopia can be automated.
MIT Media Lab director Joi Ito and President Barack Obama have commented on AI and its options, but also on the over-simplification by some of what AI can do. This five minutes video conversation with editor in chief of Wired magazine, Scott Dadich, says it succinctly.
International harmonisation of data needed
Cross-border tax transparency efforts, open procurement systems, open government data or public registers of civil servants’ interests are being introduced in several countries. Analysing the complexity by combining such datasets from different sectors or even countries demands the assistance of AI-applications simply because of the volume of data. A major obstacle to doing so is the lack of standardisation and harmonisation of data. Operating across multiple languages, currencies, and procedures increases the challenge to combine various types of information.
The World Meteorological Organization (WMO) makes sure that global weather data are collected and shared according to standards agreed upon 70 years ago by 191 countries. Partly because of this, and because the data exist in a digital format, anyone with a smartphone can check the weather on the other side of the planet in real time or very nearly so.
Financial transactions data, bids and contracts information, company registers, and public spending databases do not reside under a standard regime. But AI tools can even be set up to harmonise different sources of information to prepare the data for deeper analysis. There are supposed to be about 300 million legal entities in the world. An ongoing project by CIMA includes machine learning and semantic modeling to try to harmonise information from a plethora of company registers. The aim is to make existing registers easier to use, and to harmonise entries from public datasets, activist sources such as Wikileaks or the Panama Papers, as well as semi-commercial and commercial sources.
The introduction and continuous maintenance of open data platforms and the harmonisation of registers are important steps to create data sources that will enable the use of AI and machine learning applications to detect fraud or corruption. Financial inclusion, the digitisation of transactions such as through mobile money and development of digital government services, will also add to the options.
Meeting the challenge of access to good data
Digitisation needs to happen for the AI-revolution to take place. One cross-national aim for IBM and the company’s more than 24 national offices in Africa is to improve the amount and quality of data from various sectors of society. The lack of reliable and consistent data, for example from the off-grid economies, is a key challenge. IBM is drawing on resources from their ordinary business offices to support this digitisation effort.
Another approach is to investigate what is possible to extract from digital traces generated through the use of mobile phones. Anonymised Mobile Call Records (CDR) are sometimes released for particular purposes and can be the source for a variety of analyses. Social networks or available satellite imagery are other sources. Dalberg Data is one of the companies extracting critical information on food security from such sources, as well as from census and survey data. Predictions on crop harvests based on AI analysis of Sentinel-2 satellite imagery are used for decision making on food security. Ground truth data collected by teams visiting selected areas are identifying types of crops and harvest rates. The information is collected to calibrate (train) the AI. The same ground truth is needed to verify the outcomes of the AI predictions.
Mpesa and mobile money has enabled financial inclusion for millions and helped to reduce the cost as well as the risk of fraud and corruption in public spending. As transactions move from cash to a digital platform, they become easier to trace.
A key risk in projects utilising mobile data records is that the data you get may not be the data you need. Uncritical use of proxy data includes a severe risk for bias, in particular if minorities, rural populations, or vulnerable groups are involved. However, it should be noted that as the use of proxy data may be appreciated in one country, it may cause a scandal elsewhere.
Big data ecosystem dashboard in Uganda
Funded by the Belgian government and in collaboration with UNCDF, Dalberg Data launched and tested their platform ‘Action Insights Data‘ (AIDA) during a pilot project in Uganda in 2018. The concept is to harvest digital traces of everyday life and movements from sources such as telecom data, social media data, or transaction data in combination with data from sensors, satellite imagery, and more traditional sources such as census data or surveys. Private companies already use this kind of information to improve their products, and the idea is that advanced analysis of already existing data may be used for public good. Information is collected, compiled, and processed in near real time with AI assistance. The test in Uganda includes dashboards with information on food security, financial inclusion, and urban planning. ‘The goal is, first, to accelerate the use of private and public data sources and, second, to create an entire data ecosystem for the country, while complying to regulations and ensuring privacy,’ the company says about the project.
Cognitive AI chatbot giving verbal advice to farmers in India
The IBM-supported FarmChat research project in India may illustrate how mobile phone voice calls can act as input and output channels for an AI application. FarmChat recorded, stored, and then made public 8 million calls during a two-year period (2015–2017) to the Kisan Call Center giving farming advice in India. This dataset formed the basis for an AI research project aiming to train a chatbot to give spoken advice in Hindi to local farmers. Apart from the possible agricultural success such a project should bring, FarmChat shows how digitalisation for AI applications may be tailored to illiterate users. But, as the project reported: ‘Speech as input failed for a few of the illiterate participants (4/11) who were not able to speak Hindi fluently.’
Will artificial intelligence really lead to job loss?
Many travellers have experienced feeling insecure during taxi rides, unsure of the route taken or the price they will be charged. The high cost of renting a driver was a primary reason Garrett Camp started thinking about a networked transportation solution. His brainchild, Uber, created a commercial arena for ride-sharing in 2009. Today, the company is present in more than 170 countries, providing transportation, food delivery, and working hard to realise self-driving cars, leaving the drivers behind.
In many cities, passengers trust a ride with Uber more than they trust ordinary taxi services. All drivers are identified, every trip is rated by drivers and passengers, the cars are traced with GPS, all transactions are digital, and the deal is signed before entering the car. Strong incentives are in place to deliver a smooth ride from A to B. The procedure is less prone to cheating or fraud, passengers feel safe, and there are no disputes over the fare or the best route to take.
But the list of criticisms of the company is long. They are banned from operating in several countries, and the taxi industry is often their main opponent. Drivers are independent contractors, which leaves them few labour rights. Median wages are in some countries found to be below minimum wage, when the costs for the vehicle and insurance are subtracted.
‘Uberisation’ is a term derived from the name of the company, which describes a business model with peer-to-peer transactions between client and provider, usually omitting a centralised organisation. The model is disputed for its role in threatening ordinary jobs and for operating in a largely unregulated territory, often sidestepping labour rights and even taxation.
The last 25 years have seen the development of new ways of doing business that would be unthinkable without technology and the internet. In 2006 the book Worldchanging presented us with what the Guardian reviewer Josh Lacey called the ‘light green’ version of how to save the world. In the book, there is a short presentation of the 12 years young, but successful online bookstore Amazon. Already five years after the start, its founder Jeff Bezos ranked on the Forbes list of the wealthiest people on the planet. The reviewer stated about the book,‘it is a vision of how things might look if the geeks inherit the Earth.’
In the trail of the Amazon success lies thousands of closed local bookstores replaced by a centralised, monopolistic, AI-driven logistics company. They use contracted, low-wage delivery drivers and have the aim of swallowing ever more of the consumer goods retail market. Today’s big five, Apple, Microsoft, Google, Facebook and Amazon, have taken the dominant role in producing gadgets, in advertising, in retail, and in cloud services. Who is going to advertise on Google and Facebook when Amazon has swallowed all retailers, Conor Sen asked in a Bloomberg Opinion piece. Some of these companies are opaque, protecting themselves from outside inspection. Others challenge privacy regulations and are accused of tax evasion in countries where they skim the market for advertisements. Some of them certainly profit from their monopolies, heralded as a business strategy in recent literature such as in The Blue Ocean Strategy.
The 2016 World Development Report, dedicated to digital dividends, discusses the internet-enabled transport services disrupting over-regulated taxi markets. The report also acknowledges the risks implied when a few dominant companies have become so powerful that they can quickly buy out possible competitors to gain new markets.
The 2019 OECD report, Going Digital: Shaping Policies, Improving Lives, describes a significant rise in private investments in AI startups. Such companies gained 12% of worldwide private equity investment in during the first half of 2018.
In a discussion paper from September 2018, McKinsey Global Institute analyses the impact of AI on the World Economy. Whether companies choose to use AI ‘for innovation rather than efficiency gains’ will make a big difference on economic outcomes. If AI is used for automation of manual processes, developing countries may be the worst off, risking unemployment among thousands of employees performing tasks that automation can easily do instead.
AI has a great potential to contribute to increased economic activity on a global scale. It will happen gradually, with increased acceleration as the technology and its applications mature.
Depending on how the technology is adapted by different economies, it is considered a key challenge that AI may increase the wealth gap between countries, companies, and individual workers. Countries and companies who apply the technologies at an early stage are likely to benefit from the introduction of AI, while others will be left behind.
Increased job opportunities based on communication technologies are held up as one of the great gains AI provides. The question is if those jobs demand higher education than the jobs lost required. Education systems need to be in place to qualify workers for decent paid jobs in the digital society.
Human AI trainers wanted
Technical challenges are sometimes limiting the application of AI in business. AI applications are seldom generic, such as a word-processor. They are very specialised applications. For a machine to learn, it must normally be taught not only by programming the algorithm (formula) on which it should operate, but also by being trained on how to interpret the specific type of data to which it is applied.
The technique most common to produce value in the industry today is the machine learning variant called ‘supervised learning.‘ The technique is typically used in applications for spam detection, information retrieval, or pattern-, speech-, or character recognition.
The application is trained on a known dataset to enable high output accuracy when it is fed new and unknown data. If the machine is trained on larger datasets, its ability to deliver a correct answer increases. Still it may be a challenge to apply the system to a new, related field. A chatbot trained to understand and answer questions for an insurance company is not likely to perform well if deployed in a bank. Machines do not understand concepts nor how to apply them in new situations. They handle text, numbers, and pixels according to their programmed algorithms.
Training is often supervised by humans. In fact, AI-trainer is an up and coming profession. The job is to train corporate chatbots to understand questions and provide a correct answer for a given audience in a given language for a given company.
AI research in developing countries
Companies such as Google and IBM have established AI research centres in Ghana, Kenya, and South Africa. IBM is bringing Big Data analytics and cognitive computing to Africa with several aims. As a foundation for their investments, IBM is identifying ‘commercially viable’ projects in the fast-growing economies on the continent. The research is covering fields such as agriculture, business establishment procedures, and improving waste collection. As Jack Bright puts it in his TechCrunch piece, ‘yesterday’s development problems could be today’s commercial tech opportunities.’
IBM claims that key elements of their strategy have been to localise AI-research close to where the solutions are being deployed, to improve local competencies, and to support project development for business, governance, health, and agriculture. The company has been present in Africa for more than 50 years. IBM sees business opportunities in the fast growing economies in the global south and hope for ‘double-digit growth by providing the infrastructure citizens and government partners require.’
Dalberg, the company involved in Uganda as described above, has also been involved in the digital ID project Aadhaar Initiative in India for more than ten years. Taylor and Broeders in Geoforum question this project for the possible complications arising from its being ‘the largest-scale public–private partnership currently underway in a developing country in terms of its coverage of the population.’
In 2018 Google announced the opening of an AI research centre in Accra, Ghana. The company intends to be ‘working with policy makers on the potential uses of AI in Africa… in areas such as healthcare, agriculture, and education.’
Google, DeepMind, and IBM, among several other AI-related companies, are involved in education, networking, and the support of AI capabilities and competencies among African researchers through the project ‘DeepLearning Indaba.’ In 2019, the IndabaX meetups are being held in 26 countries across Africa. Different formats are used, such as one-day meetings, lecture series, a workshop, or a hackathon on a specific subject. The Indabas are supported financially by sponsors and are aimed at strengthening local hubs of machine learning communities across the continent.
Big players with an ‘AI for good’ approach
IBM researchers are dedicating resources to advance their AI technology and to scale the application of their solutions. They have devoted particular effort to addressing various aspects of trust in AI. Security challenges in large systems handling sensitive information is one area of research, while explainability and lineage are others. The problem of bias in machine learning models is a significant obstacle for a general trust in such systems. IBM has met this challenge by developing a dedicated, open source toolkit, AI Fairness 360, to analyse the models, identify problems, and mitigate them. The tool covers issues in credit scoring, biased predictions, and challenges connected to equal opportunities for privileged and unprivileged groups.
We already mentioned the PAIR initiative and the counterfactual testing tool for algorithms. Google also flags its AI for social good to address global challenges in health, education, and natural resource management. And data-driven philanthropy is the aim of Google.org, where grants to organisations and projects are supported by employee volunteer hours for good causes. Global education and job opportunities are particular areas of interest.
AI for earth, AI for humanitarian action and AI for accessibility are three areas where Microsoft AI for good channels the company’s support. The company has developed their approach to ‘good’ by launching the thematic areas successively.
Lastly, Partnership on AI gathers more than 80 organisations ‘on a mission to shape best practices, research, and public dialogue about AI’s benefits for people and society.’ Civil society organisations, startups, and academic research institutions are represented along with major for-profit players in the field. The work is organised as six thematic pillars covering safety, transparency, fairness, AI’s effect on labour and its societal influences, as well as AI for social good. By engaging experts and stakeholders, the organisation enables studies and research by independent third parties. Partnership on AI also supports ‘aspirational projects’ and the development of learning materials to promote best practices. So far, no partners from Africa or South America are listed.
Information capitalism
There are critics of distance sensing and remote management, and those critical voices are also concerned about the intimate connections created between NGOs, donor agencies, commercial companies or foreign state agencies. Mark Duffield claims that ‘Learning how to make money in the sub-prime tele-economic conditions of the global South is the private sector’s last global frontier.’
Other critics question the increased collection of critical statistical data by corporations. Linett Taylor and Dennis argue that there is a shift from states collecting data, identifying, and categorising individuals and whole societies to corporations taking over the collection and analysis. These critics therefore investigate ‘the implications of seeing development interventions as a by-product of larger-scale processes of informational capitalism.’
It is not for this report to go in-depth on how private enterprises gain power as they engage with governments in developing countries. But there is a likelihood that the introduction of ever more digital technology may increase the interest in investing in the global south. Are the ‘AI for good’ programmes by multinational companies a camouflage for hard business?
Multinational tech companies support competence development in developing countries. Development agencies sometimes join as partners, with the aim to lift people out of poverty. The commercial potentials in these collaborations should not be ignored. Digital information on all aspects of society or social life are valuable commercial assets for new business concepts. NGOs or donor agencies risk getting carried away by the digital hype in ‘cool’ artificial intelligence projects for which funding also might be easier to achieve.
A ‘tech startup-boom’ is occurring across the developing world where multinational tech-giants, development agencies, and financial institutions join forces to stimulate modern businesses, preferably utilising disruptive technologies. Ory Okolloh, the co-founder of Ushahidi, calls it a ‘startup-fetishism’ rolling over the African continent. Under conditions in which many lack electricity, drive on bumpy roads, and have weak governance systems, ‘who is gaining from the growth,‘ she asks in an interview in The Nation/LeMonde Diplomatique. ‘You can’t entrepreneur around bad leadership, we can’t entrepreneur around bad policy,’ Okolloh says.
One may ask if that is exactly what IBM research is attempting to do in the collaboration with the Kenyan government to improve the country’s ranking on the ease of doing business list. The project claims to be successful, yet its full effects are unknown. One of the effects should be to gain tax funds for investment in public goods, fixing the bumpy roads and strengthening the power grid.
Encountering the black box problem
This report addresses the prospects of AI for anti-corruption. Still, as it contains examples of how existing governance procedures can be replaced with AI-driven applications to omit previously corruption prone procedures, we should spend a few lines on current discussions about how such algorithms are governed.
There are several concepts of how artificial intelligence applications approach a problem. As mentioned by Andrew Ng above, the model where a complex formula, the algorithm, calculates single or multiple streams of inputs to deliver a specific output is a simple, but for the purpose of this discussion, a functional way of describing how AI applications work.
The algorithm may consist of millions of lines of code. In the algorithm framework called Neural Networks, several algorithms are combined in sequences to perform calculations. Their complexity can make it impossible to tell exactly how the calculation resulting in a given output is performed. It is not an option to go back in the formula to track down which attribute of an input led to the output. This problem is what is called the ‘Black Box problem.’
In other words, the process between input and output is obscure. The simplified illustration ‘Data inputs –>Algorithms–>Data output’ is apt to describe the process, even if the code within the algorithm is hidden or too complex to explain.
The code inside ‘the box’ may also contain protected intellectual property and business secrets, which is another reason not to reveal the way it works. As the use of AI applications increase, so does the risk for algorithms being used intentionally or not, for manipulation, censorship, or discrimination. Hence the need for governance of the processes inside the machine. How to do this in practise, however, is unclear and inconclusive.
Many countries lack the legislation to govern the behaviour of non-human decision systems. When such systems are put to work to make decisions in society, the ‘Black box problem’ can hinder the ability to contest the machine’s decision. Decisions on pensions, financial credibility, or the result of bids in a procurement process should be possible to explain and dispute.
The ‘right to explanation’ as it is expressed in the European General Data Protection Regulation (GDPR) is not satisfying, claimsJavier Ruiz in the Open Rights Group. He claims that the narrowly defined right is ‘not compatible with how modern machine learning technologies are developed,’ and therefore needs a significant update to cover the new realities.
Machine Learning has demonstrated its capabilities and is likely to become even more a part of our ‘critical societal infrastructure,‘ as Lillian Edwards and Michael Veale put it. Rather than demanding transparent algorithms, they argue that the right to be forgotten (right to erasure) and privacy by design might be the starting point to develop responsible, human-centred machine learning systems.
Researchers at the Oxford Internet Institute and at The Alan Turing Institute suggest the use of counterfactual processes to contest what goes on inside the algorithmic ‘black box.’ Such processes do not affect the code as such, but they challenge the outputs by investigating how different inputs would alter the outcome. As this method investigates external inputs and outputs, the internal functions of an algorithm can be left alone. ‘As a result, counterfactuals serve as a minimal solution that bypasses the current technical limitations of interpretability, while striking a balance between transparency and the rights and freedoms of others (e.g. privacy, trade secrets).’
From a similar angle, the internal initiative at Google, the People + AI Research (PAIR) group has, among other initiatives, developed the What-if Tool to enable the evaluation and comparison of different machine learning models to improve performance and fairness. The tool allows for testing algorithm outcomes by altering inputs and editing datapoints in a given model.
Stephen Cave, director of the Leverhulme Centre for the Future of Intelligence at the University of Cambridge fears that biased algorithms will ‘perpetuate existing forms of discrimination, and even exacerbate them.’ He refers in this comment in The Guardian to Frans Kafka’s novel The trial to exemplify worst outcomes. Cave shows how an opaque AI system based on personal data, making decisions over people’s lives, without those people knowing which information the system possesses and how it does its reasoning, resembles the helplessness of Josef K. towards the system that arrested him. Steven Cave concludes his concern by stating: ‘Those who have historically been failed by systems of power, such as Kafka – a German-speaking Jew living in Prague – have always been particularly well-placed to recognise their opacity, arbitrariness and unaccountability. Including those voices will therefore ensure that AI makes the future not just more efficient but also more ethical.’
Taking a look at the staff page presenting the team behind a contemporary AI start-up can be a good starting point to ask if such voices are included in the development of these powerful tools.
Even in our highly digitised societies, we struggle to keep up with regulations, laws, and responsible governance of a rapidly developing industry. The implementation of GDPR in 2018 is an example of a much needed regulation finally agreed upon and implemented. In countries with weaker regulations, it is likely that ‘commercially viable’ solutions will be developed which may or may not comply with ethical standards in the home country of the company developing them. It would be the responsibility of a donor organisation to ensure that supported projects include compliance with international ethical standards.
Who wants to be governed by an algorithm?
The Chinese approach to apply AI and surveillance for monitoring its citizens to see if they are behaving as they should creates headlines. The government has installed millions of surveillance cameras, deployed face-recognition AI and score systems punishing bad behaviour and encouraging loyalty to the state and good habits in several provinces of the country. Many fear that the Chinese model of algorithmic governance will become a real digital dictatorship. Nick Bostrom and his Vulnerable World Hypothesis is one of the researchers deeply concerned about such a possible dystopian future. Arguably, such an application of AI goes a step further than the persuasive technology embraced by western societies. Accordingly, perhaps a short reflection on how behaviourism, data-collection and nudging is embedded in our gadgets would be warranted here for purposes of comparison.
Persuasive Technology or ‘Captology’ is a term coined by MIT researcher B.J. Fogg at the turn of the millennium. The word describes computer-assisted technology and gadgets designed to make us behave in a desired way. A pedometer on the wrist or embedded in a mobile phone persuade us to walk a minimum daily distance. Radar-enabled signs along the road prevent us from speeding simply by telling us how fast we drive. Some of these tools may record our mistakes and result in penalties, such as ‘dots’ on the driver’s license.
Other interactions with algorithms are more subtle. Machines suggest which movie to watch, whom to invite for a date, or which book to purchase. Therapy chatbots act as psychological advisors, while others can act as personal financial guides.
How to think about such technologies in relation to anti-corruption or fraud prevention may be relevant. It is a different approach to choose the path of surveillance – such as in the above described ‘Project Insight,’ or to develop behavioural design ‘nudging’ towards a desired behaviour. Somewhere in between the two positions there is a fine line that we do not want to cross.
If all datasets are biased, the AI will make biased decisions
Another pitfall is to believe in unbiased data. All data to date has inherent biases. Thus, it is fairly simple to explain the biased outcomes of algorithms. An example can be visualised on the algorithms driving Google Translate. The tool is trained on an already existing corpus of text, reflecting historical states as well as the current state of affairs. Translating from Turkish, which has no grammatical gender, logically leads to gender-biased translations of specific phrases. The history of gender bias is confirmed in the results. Typing ‘o bir doktor‘translates in English to ‘he is a doctor.’ Writing the phrase ‘o bir hemşire’ translates to ‘she is a nurse.’ To compensate, Google has introduced a tool for gender-specific translations. If this tool is applied, the output for ‘o bir doctor’ comes in a female and male version. However, the user who improves a translation must actively select the tool, which may not always happen. This way, the translation tool perpetuates historical bias.
Algorithms are deciding which posts to boost and which ones to suppress on Facebook. Similar formulas govern which videos get promoted. Certain criteria including number of likes or how often a message is shared are included to calculate content promotion. The opaque decision-making system behind this calculation is criticised for partly contributing to the polarisation of online debate.
Echo-chambers where like-minded users share and boost their views are not only affecting society in the US or Europe, but are also identified as a significant contribution to the polarisation and escalation of conflict levels in Egypt as well as Myanmar. Organisations like PeaceTech are systematically fighting hate-speech in social media, and it should be no surprise that artificial intelligence also is applied in the battle.
Several states in the US have begun assessing the risk of convicts' reoffending based on personal and demographic data driving algorithms to decide the regime under which the offender is to be rehabilitated. In theory, such a tool should reduce bias in decisions made by individual judges – since judges apparently they make different decisions depending on the time of day, or whether the judge is hungry or tired. The AI-driven system turns out to be based on data with a similar risk of bias and may in fact send people in jail if the statistics end up giving the convict a high score. Statistical information on population demographics, covering education, race, income, and area of residence can generate severe bias when used in AI-driven decision making systems, as is seen, for example, in predictive policing.
Mobile phone data from a country where owning a mobile means you are likely to be urban, male, and wealthy are already biased. The dataset showing the movement of these phones every morning are perfect to apply for planning public transportation for the wealthier half of the population in a poor country’s capital. But the dataset will likely generate bias if used for purposes reaching rural populations, women, or children. Nathan Begbie argues that we should differentiate between biased datasets. He contends that it is not necessarily bad to differentiate between rich and poor, between a credit-worthy groups and those who are not. The problem arises when the algorithm discriminates on an illegitimate or discriminating basis.
To mitigate bias and errors, algorithms must be tested in different contexts, and monitored during usage. In donor-driven projects, the use of what might have been an expensive but malfunctioning system has to be avoided. When Amazon dismantled their infamous AI recruitment tool in 2018, they proved that their system had become problematic in just this way. The system had been trained on thousands of previous job applications and their outcomes, and was abandoned due to its bias towards prioritising applications from male candidates.
There is a severe risk included in introducing decision-making systems which are super-efficient, cost-saving, and rational. Their decisions may be hard to dispute, may contain biased decisions or discriminate against particular groups. Such effects may not easily be recognised before the system is actually launched. In a development context, where proxy data frequently are used due to the lack of survey, census, or other demographic data, the risk of biased datasets for the training of algorithms is high and therefore needs particular attention.
Ethical guidelines from different standpoints
We are in the middle of rapid development of AI-technologies. Researchers, human rights organisations, and workers’ unions are focusing on the ethical sides or side-effects of applying AI-technologies to ever new fields. We need to ‘…formulate foresight methodologies to indicate ethical risks and opportunities and prevent unwanted consequences,’ says Mariarosaria Taddeo and Luciano Floridi in their piece in Science on how AI can be a force for good. Important institutions have published guidelines or ethical principles. Below are extracts from a few of them.
- The Institute of Electrical and Electronics Engineers (IEEE) is developing Ethically Aligned Design, a set of policies and guidelines ‘in order for such systems to remain human-centric, serving humanity’s values and ethical principles.’ Privacy International is one of the organisations worrying about the risks involved in the massive spread of AI-driven products and systems. Large amounts of data are collected without consent and compiled to profile individuals. Tracking of individuals, discrimination, opaque decision-making, and exploitation of personal data are just a few concerns. PI calls for inclusion of human rights principles in the development of AI projects. Laws and regulations need to be reviewed and amended to protect individuals from ‘new and emerging threats to privacy.’
- UNI Global Union represents 20 million workers from over 150 countries. The 10 Principles for Ethical Artificial Intelligence is a guide for workers unions to raise their voice as AI enters workplaces all over the world. The organisation is calling for action to safeguard workers’ interests and maintain a healthy balance of power in workplaces.
- The Scientific Foresight Unit of the European Parliament has published a brief on Legal and ethical reflections concerning robotics. It expresses concerns over risks to human safety, privacy, integrity, dignity, autonomy, and data ownership, and asks if current legislations can cope with the expected legal challenges AI and robotics will bring about.
- Rights to equality and non-discrimination in machine learning systems are held in the forefront of the Toronto Declaration. It was drafted by members of different human rights and digital rights organisations, including Amnesty International and Privacy International. The declaration identifies both state and corporate obligations to defend human rights in machine learning projects.
The above briefs and guidelines cover AI technology that is available today and applied in all sorts of new products, services, and governance. But a significant body of work also raises a deep concern over the possible futures of AI. Max Tegmark is one researcher who brings together the most qualified thinkers to debate the future of technologies such as AI, through the Future of Life Institute.
- Concerned for the future developments of AI, the Asilomar principles suggest that the goal of AI research should not be to develop an ‘undirected intelligence,’ but rather ‘beneficial intelligence.’ The guidelines seek collaborations between AI researchers and policy makers, and emphasise equality, humanity, and rights. About 5,000 signatures support the principles, which are meant to be overarching, global guidelines for what may lead to the development of truly intelligent machines.
- The Barcelona declaration for the proper development and usage of artificial intelligence in Europe distinguishes between knowledge-based AI where the foundation comes from ‘conceptual models, reasoning and problem solving strategies, language processing, and insight learning.’ Data-driven AI on the other hand, ‘starts in a bottom-up fashion from large amounts of data, which are processed with statistical machine learning algorithms, … in order to abstract patterns that can then be used to make predictions, complete partial data, or emulate behaviour based on human behaviour in similar conditions in the past.’ The combination of the two will release the full potential of AI, and the declaration seeks to contribute to the ‘proper development and usage’ of AI in Europe and to become a code of conduct for AI practitioners – both users and developers. Similar to the Asilomar principles, the Barcelona Declaration is open for signatures.
Recommendations
- Clearly define the problem for which AI can be applied in reducing corruption in development cooperation
- Partner with diverse competencies where technologists are supported by ethical and social capacities.
- Ensure local ownership and inclusion in processes and management of data.
- Support education and research on site. Include the voices from where the projects are meant to operate.
- Develop and nurture digital literacy in donor agencies, within governments, in NGOs businesses, and among citizens.
- Ensure the inclusion of the illiterate, marginalised, and poorest segments of populations.
- Strengthen and utilise cross-organisational entities for technical advisory, ethical, or legal support to improve competencies of organisations, donors, and practitioners in the rapidly developing field of AI.
- Apply a holistic approach in designing new projects, and acknowledge that:
- AI is not solving corruption on its own no matter how effective it may be in predicting or revealing misconduct or abuses.
- AI will lead to changes in the labour market where some jobs will become obsolete and new professions will appear, most likely with a higher demand for qualifications.
- The implementation of powerful technologies demands ethical considerations and risk evaluations.
Appendix
Machine learning, artificial intelligence or superintelligence?
To put things very simply, we can say that artificial general intelligence (AGI) or superintelligence is computer intelligence with the aim to match human intelligence. Still a construction belonging to the (far) future, it refers to a machine that is able to think and reason.
The artificial intelligence applications available today do not really understand what game they play, what images they see, or what music they suggest. All they do is to (cleverly) categorise input information to generate an output or an action. The information may be text, numbers, pixels, or digitised sensor data. The great advantage of machines is that they are able to analyse vast amounts of data from multiple sources incredibly quickly and by this, simulate intelligence. An algorithm is the mathematical ‘formula’ by which the calculations are done and may contain a million lines of code or more. The output can be text, synthetic voice, sound, or signals. When these are triggering physical movement, some would call that a robot.
There is no real consistency in the use of the term ‘artificial intelligence’ and ‘machine learning’ in this text or in the references we have used. However, a simple and understandable description is found in the USAID report, Reflecting the Past, Shaping the Future: Making AI Work for International Development.
Data consist of text, numeric information, images, audio, or video. This is the input step.
Machine learning represents the second step and the methods used can be supervised learning, unsupervised learning, reinforcement (trial and error learning), or deep learning. The latter is what comes into play for image recognition, diagnosis applications, and other complex tasks.
Artificial intelligence is often used as a general description of the whole system, resulting in chatbots, computer vision, or decision systems.
Further descriptions of the concepts in plain non-tech language can be found in this beginners guide from IBM.
The recommendations above aim to 'develop and nurture digital literacy' within organisations and donor agencies planning to engage in AI. One NGO who has specialised in such needs for the development sector is Techchange, offering a wide spectre of online education.
We have included a few references to the research on AGI, because ethical questions concerning the more advanced technologies possibly to come may be relevant also for other applications of AI. Artificial intelligence in our context is about the ability to use computing power to solve complex computations or to analyse of large sets of data. Such goals may be to extract relevant information from large, static datasets or streams of data. It may be to predict the likelihood of a given outcome, or to identify anomalies. AI applications are able to decide outcomes on the basis of embedded rules (algorithms).
Datasets too large for humans to sift through easily could be data from financial transactions, procurement history, or how social or business networks are connected and affect other pieces of data. Imagery or non-structured data from social media or meta-data from various communication platforms may also be used as inputs. Information may come from sensors built into objects such as ships, cars, mobile phones, or implants. Artificial intelligence, machine learning (ML), big data, the internet of things (IoT) and blockchain technology are in some instances closely linked together and included in some of our examples.
AI and machine learning may be used to harmonise, categorise, or sort large datasets to select the records interesting for further investigation. The data may be a stream of input such as continuous transaction data, analysed in real time to flag suspicious transactions, or it may be millions of data-records sorted for detailed inspection, for example by tax authorities.